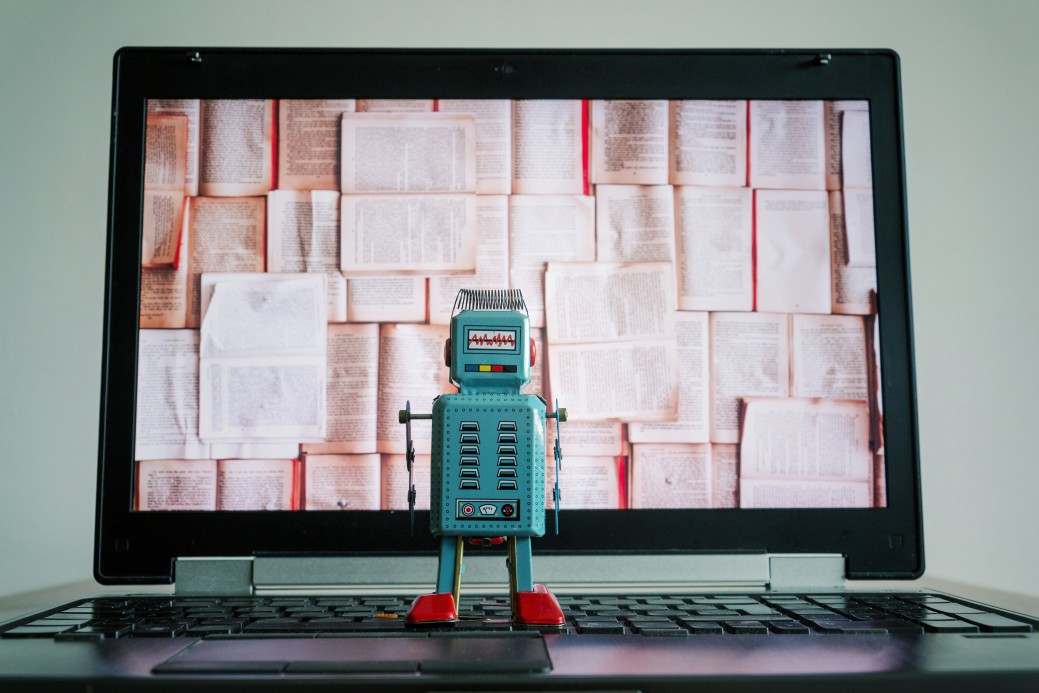
As advanced enterprise automated machine-learning platforms continue to evolve, data scientists are increasingly the hero figure of their organizations. From processing large volumes of a variety of numbers, such as operational and customer data, to credit data, consumer research, and third-party data sets, data scientists empower management and key stakeholders to make enhanced decisions with quantifiable, data-driven evidence. They’re even able to wrangle in and make sense of the typically chaotic data born of social media streams.
With flexible hybrid data as a service (DaaS) and big data as a service (BDaaS) solutions, data scientists are able to access and process data on hosted solutions. This grants potent, real-time insights while also maintaining control and privacy of their exclusive on-premises data. With that, data scientists have had an easier time of controlling costs, eliminating certain advanced-data-analytics investments, including a range of business intelligence (BI) technologies and applications.
Despite enhanced machine-learning capabilities, new analytics tools, increased processing power, and stronger support infrastructures, data scientists still face mammoth challenges. The following discusses three of the top pain points of data science.
1. Too Much Post-Mortem and Not Enough Predictive Analytics
A majority of analytics today focuses on post-mortem examination, investigating old data to assess past events and why they happened. Descriptive analytics are used to guide future actions. For example, post-mortem data highlights helpful customer information such as gender, which particular product or service they bought or are subscribed to, and how many touch points they’ve had with customer service or account management. This allows businesses to build detailed customer profiles. With that data, for example, organizations can better decide on promotions for particular customers to reduce churn and improve retention.
However, descriptive analytics often fail to provide helpful customer data in a timely manner. Chances are a client who has churned out of an organization’s system and is now with their competitor because they weren’t properly serviced, optimally marketed, or upsold to, etc. In other words, customer profiles are only beneficial if you’re able to identify a customer as having that profile at the time you’re talking to them. That’s why predictive analytics are so important.
Predictive analytics allows businesses to analyze the profiles that most often lead to churn, enabling them to act fast and retain more clients. You gain the capacity to identify the specific actions, transactions, touchpoints, and interactions that have a high probability of producing a specific outcome such as customer churn. Predictive analytics uncover these churn indicators in real or near-real time to tell you more accurately how and when to act.
2. Untouched Values and Inefficient Data Usage
Data analytics methods and tools too often analyze only numerical data, failing to investigate categorical values. Many organizations fail to recognize the value of fusing disjointed data, chaining together siloes to enhance cross-department communication and functionality. Without fused and normalized data, organizations are unable to extrapolate one department’s variables and apply to others. Comprehensive, collaborative data science has the ability to unearth universal variables that can be translated across departments to more efficiently optimize KPIs.
Free-form text and unstructured data represents another land of missed opportunity. Organizations fail to mine the gold that’s waiting to be found in email, social media, as well as customer service, account management, and sales calls.
3. Insufficient Accessibility and Expertise
Most organizations will agree that one of their top challenges is the retention of their data scientists. They’re one of the most sought-after, headhunted positions throughout data-driven industries. Organizations are often victim of vicious train-leave cycles, getting them up to speed on their unique operations just in time for them to be stolen for higher pay by a competitor.
And while today’s data science tools and platforms offer increasingly greater capabilities, even the drag-and-drop options require increasing data-science expertise to actually leverage solutions to their full potential.
Therefore, the combination of data-scientist-retention challenges and the growing data-science expertise needed to leverage top analytics tools has proven to be an intense problem for organizations.
To address the problem of data-scientist retention, more organizations are plugging their department managers into the equation. Yes, department managers are among the endpoints who need to actually put the data into action, applying it to their marketing, advertising, customer service, and operations decisions. But they simply don’t have the expertise required to adequately operate today’s increasingly complex data-science tools and platforms.
Comprehensive collaboration between experienced data scientists and department managers is the best approach. But since that’s not always an option, ease of use with data science tools is increasingly important. This has made intuitive web-based interfaces indispensible for countless organizations. With enhanced user friendliness, built-in data visualizations, and more flexible evaluation models, organizations are able to get the most from their in-house resources and make better sense of the data chaos flowing throughout and around their business.
HOW CAN WE HELP YOU?